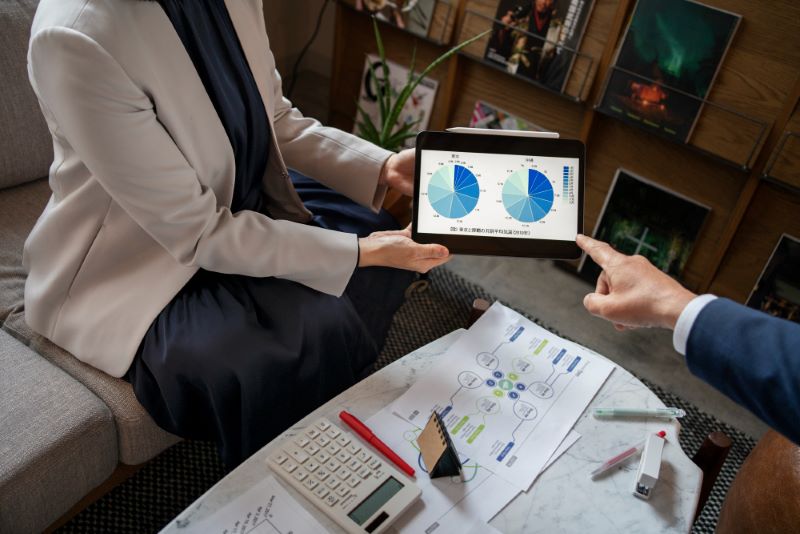
Introduction
In today’s fast-paced retail landscape, staying ahead of the curve is not just a competitive advantage; it’s a necessity. As the industry becomes increasingly data-driven, retailers are turning to advanced technologies to anticipate market trends, manage inventory, and provide a personalized shopping experience. One of the most transformative tools in this evolution is retail predictive analytics. By leveraging historical data, machine learning, and AI, retail predictive analytics allows businesses to make informed decisions that drive profitability and customer satisfaction.
The Basics of Retail Predictive Analytics
Retail predictive analytics is a technique that uses statistical algorithms and machine learning to identify patterns in historical data. This data is then used to predict future outcomes, enabling retailers to make data-driven decisions. The applications of predictive analytics in retail are vast, ranging from demand forecasting and inventory management to personalized marketing and customer segmentation.
Why Retailers Need Predictive Analytics
The retail industry is notorious for its slim profit margins and fierce competition. To succeed, retailers must balance inventory levels, optimize pricing strategies, and enhance the overall customer experience. Retail predictive analytics provides actionable insights that can help retailers:
- Forecast Demand: By analyzing past sales data and current market trends, predictive analytics helps retailers anticipate demand fluctuations, reducing the risk of stockouts or overstocking.
- Optimize Inventory: With accurate demand forecasts, retailers can manage their inventory more effectively, ensuring that the right products are available at the right time.
- Enhance Customer Experience: Predictive analytics can be used to personalize the shopping experience by recommending products based on customer preferences and purchase history.
- Improve Pricing Strategies: By analyzing competitor pricing and customer behavior, retailers can optimize their pricing strategies to maximize profitability.
Applications of Retail Predictive Analytics
- Demand Forecasting
One of the most common applications of retail predictive analytics is demand forecasting. By analyzing historical sales data, seasonal trends, and external factors such as economic conditions or weather, retailers can predict future demand with a high degree of accuracy. This enables them to plan their inventory, staffing, and marketing efforts accordingly.
For example, a clothing retailer can use predictive analytics to forecast demand for winter coats. By analyzing past sales data and weather patterns, the retailer can determine how many coats to stock in each store, reducing the risk of both stockouts and excess inventory.
- Inventory Management
Effective inventory management is crucial for any retailer. Overstocking ties up capital and increases storage costs, while stockouts lead to lost sales and dissatisfied customers. Retail predictive analytics helps retailers strike the right balance by providing insights into optimal inventory levels.
For instance, a grocery store chain can use predictive analytics to manage perishable goods. By analyzing historical sales data and considering factors such as upcoming holidays or local events, the store can adjust its inventory levels to minimize waste and maximize sales.
- Personalized Marketing
In the age of personalization, customers expect tailored shopping experiences. Predictive analytics allows retailers to create personalized marketing campaigns by analyzing customer data, such as purchase history, browsing behavior, and demographics. This enables retailers to target the right customers with the right offers at the right time.
A beauty retailer, for example, can use retail predictive analytics to send personalized product recommendations to customers based on their past purchases and preferences. This not only enhances the customer experience but also increases the likelihood of repeat purchases.
- Customer Segmentation
Retailers can use predictive analytics to segment their customer base and tailor their marketing efforts accordingly. By analyzing customer data, retailers can identify different segments based on factors such as buying behavior, demographics, and preferences. This allows them to create targeted marketing campaigns that resonate with each segment.
For example, a home improvement store can use predictive analytics to identify a segment of customers who frequently purchase gardening supplies. The store can then target this segment with personalized offers and promotions, increasing customer loyalty and driving sales.
- Optimized Pricing Strategies
Pricing is one of the most critical factors influencing a customer’s purchasing decision. Retail predictive analytics can help retailers optimize their pricing strategies by analyzing competitor pricing, market trends, and customer behavior. By setting the right price at the right time, retailers can maximize their profit margins and stay competitive.
For instance, an online electronics retailer can use predictive analytics to adjust prices in real-time based on competitor pricing and customer demand. This ensures that the retailer remains competitive while maximizing profitability.
The Future of Retail Predictive Analytics
As technology continues to evolve, the applications of retail predictive analytics will only expand. With the rise of AI and machine learning, predictive models will become even more accurate and sophisticated, providing retailers with deeper insights into customer behavior and market trends.
Moreover, the integration of predictive analytics with other advanced technologies such as the Internet of Things (IoT) and blockchain will further enhance its capabilities. For example, IoT devices can provide real-time data on inventory levels, while blockchain can ensure the transparency and security of supply chain data.
In the future, we can expect predictive analytics to play an even more significant role in areas such as:
- Supply Chain Optimization: By analyzing data from across the supply chain, retailers can optimize everything from procurement to logistics, ensuring a more efficient and cost-effective operation.
- Customer Lifetime Value (CLV) Prediction: Predictive analytics can help retailers identify high-value customers and develop strategies to retain them, ultimately increasing their CLV.
- Omnichannel Retailing: As more retailers adopt an omnichannel approach, predictive analytics will be crucial in ensuring a seamless and personalized customer experience across all channels.
Challenges and Considerations
While the benefits of retail predictive analytics are clear, implementing it effectively comes with challenges. Retailers must ensure they have access to high-quality data, as inaccurate or incomplete data can lead to faulty predictions. Additionally, retailers must invest in the right technology and expertise to analyze and interpret the data.
Another consideration is the ethical use of data. As retailers collect more customer data, they must ensure that they are transparent about how this data is used and that they comply with data privacy regulations.
Conclusion
In an industry as dynamic and competitive as retail, the ability to anticipate trends and make data-driven decisions is invaluable. Retail predictive analytics offers retailers a powerful tool to optimize inventory, personalize customer experiences, and improve profitability. As technology continues to advance, the role of predictive analytics in retail will only grow, making it an essential component of any successful retail strategy.
By embracing retail predictive analytics, retailers can not only stay ahead of the competition but also deliver a superior shopping experience that keeps customers coming back.