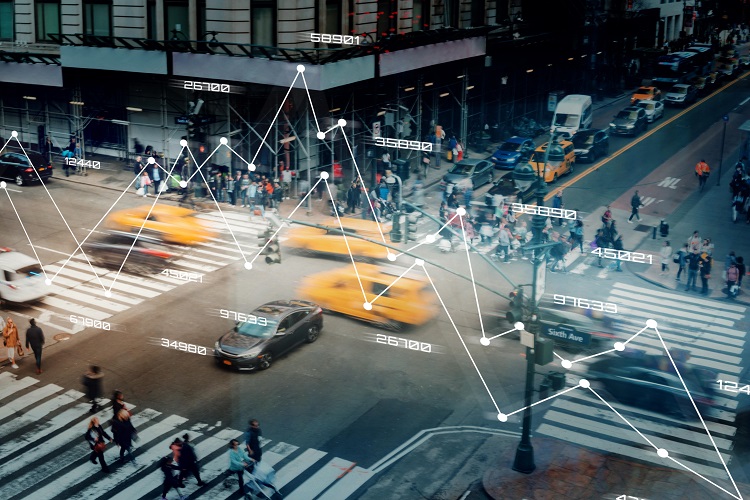
In today’s digital age, network security is more critical than ever. As cyber threats become increasingly sophisticated, organizations must employ advanced techniques to safeguard their systems. Data science has emerged as a key player in detecting network intrusions, offering powerful tools and methodologies to identify and mitigate security threats. This blog explores how data science is revolutionizing network intrusion detection and the value of a data science training in mastering these techniques.
The Importance of Network Intrusion Detection
Network intrusion detection involves monitoring network traffic for signs of unauthorized access or malicious activity. With cyberattacks becoming more frequent and complex, traditional security measures are often insufficient. Data science enhances intrusion detection by applying advanced algorithms and machine learning techniques to analyze vast amounts of network data. By identifying patterns and anomalies, data science can detect potential threats more accurately and quickly.
A data science certification provides essential training in the techniques and tools used in intrusion detection. Courses cover data analysis, anomaly detection, and machine learning, equipping professionals with the skills needed to develop effective security solutions. Understanding these concepts is crucial for staying ahead of evolving cyber threats and protecting organizational networks.
Machine Learning Algorithms for Intrusion Detection
Machine learning algorithms are central to modern intrusion detection systems. These algorithms analyze network traffic and identify patterns associated with malicious behavior. Common techniques include supervised learning methods, such as decision trees and support vector machines, and unsupervised methods, like clustering and anomaly detection.
A data science course teaches how to implement and tune these algorithms for intrusion detection purposes. Professionals learn how to train models on historical data, evaluate their performance, and adjust parameters to improve accuracy. Mastery of these techniques allows for the development of robust systems capable of detecting various types of intrusions, from known attacks to novel threats.
Anomaly Detection in Network Traffic
Anomaly detection is a critical aspect of intrusion detection, focusing on identifying unusual patterns in network traffic that may indicate a security breach. Data science enhances anomaly detection by using statistical methods and machine learning to analyze network behavior and flag deviations from normal patterns.
In a data science course, professionals gain expertise in anomaly detection techniques, including statistical methods like Gaussian Mixture Models and machine learning methods such as Isolation Forests. Understanding how to apply these techniques effectively enables the creation of systems that can detect both known and unknown threats based on deviations from expected network behavior.
Integrating Big Data with Network Security
The volume of data generated by network activity is immense, and handling this data effectively is crucial for detecting intrusions. Data science provides tools for managing and analyzing big data, allowing for real-time analysis of network traffic and faster detection of potential threats.
A data science course covers big data technologies and techniques, including distributed computing frameworks like Apache Hadoop and Spark. These skills are essential for processing large volumes of network data and extracting actionable insights. By leveraging big data tools, professionals can build systems that scale with the increasing complexity and volume of network traffic, improving overall security.
Real-Time Intrusion Detection and Response
Real-time intrusion detection is vital for minimizing the impact of security breaches. Data science facilitates real-time monitoring and analysis of network traffic, enabling rapid detection and response to potential threats. Techniques such as real-time analytics and automated response systems are used to address security incidents as they occur.
In a data science course, professionals learn how to implement real-time data processing pipelines and develop automated alert systems. These skills are critical for creating responsive security solutions that can promptly address network intrusions and reduce potential damage. Real-time capabilities enhance the effectiveness of intrusion detection systems by providing timely information and enabling swift corrective actions.
Data science has become an indispensable tool in the fight against network intrusions, offering advanced techniques and methodologies for detecting and mitigating cyber threats. From machine learning algorithms and anomaly detection to big data integration and real-time response, data science enhances network security by providing more accurate and efficient solutions.
For those interested in mastering these techniques, a data science course offers valuable training in the skills needed for effective intrusion detection. Courses cover essential topics such as data analysis, machine learning, and big data technologies, equipping professionals with the knowledge to develop robust security solutions.
As cyber threats continue to evolve, the role of data science in network intrusion detection will only grow in importance. By investing in data science education and applying these techniques, professionals can contribute to stronger, more secure networks and stay ahead of emerging threats. The integration of data science into network security practices is essential for protecting organizations from increasingly sophisticated cyberattacks and ensuring the integrity of their digital infrastructure.