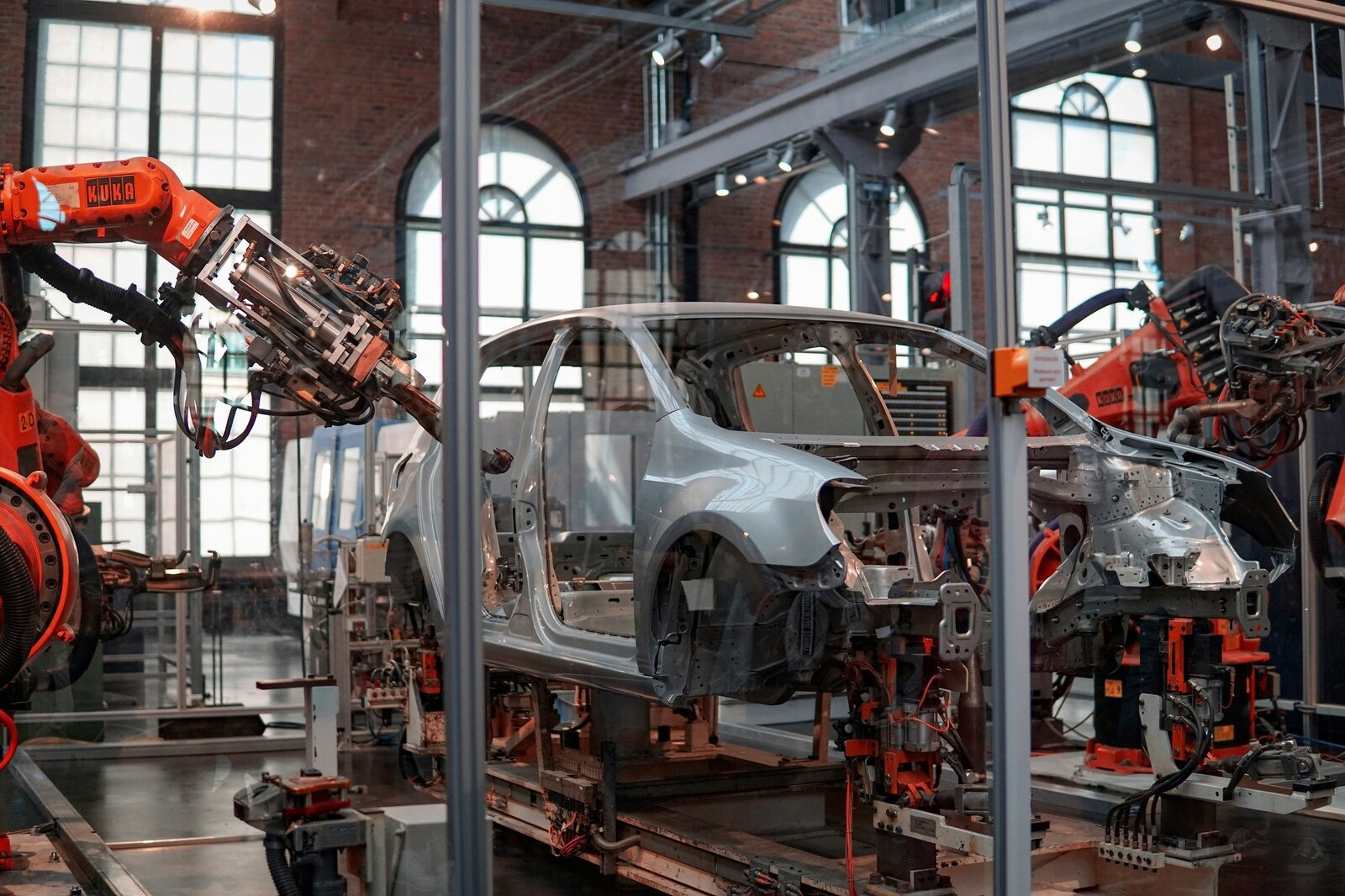
In the era of artificial intelligence (AI), data quality is the bedrock upon which successful AI systems are built. However, ensuring high-quality data for AI applications is a complex and labor-intensive process, often involving manual assessment and cleansing of datasets. This is where automated data quality profiling emerges as a powerful tool, revolutionizing the way organizations prepare and analyze data for AI. By automating the detection and remediation of data quality issues, automated data quality profiling accelerates AI workflows, enhances model performance, and drives innovation across industries. In this comprehensive guide, we’ll delve into the intricacies of automated data quality profiling, exploring its significance, benefits, and practical applications in accelerating AI.
Definition of Terms:
1. Automated Data Quality Profiling: Automated data quality profiling refers to the process of automatically analyzing and assessing the quality of datasets, identifying inconsistencies, anomalies, and errors that may impact the accuracy and reliability of AI models.
2. Artificial Intelligence (AI): Artificial intelligence is a branch of computer science that focuses on the development of intelligent systems capable of performing tasks that typically require human intelligence, such as learning from data, making decisions, and solving complex problems.
3. Data Preparation: Data preparation involves cleaning, preprocessing, and transforming raw data into a format suitable for analysis and modeling. It encompasses tasks such as data cleaning, feature engineering, and normalization.
4. Data Understanding: Data understanding refers to gaining insights into the characteristics, structure, and patterns of data through exploratory data analysis and visualization techniques. It helps data scientists comprehend the underlying relationships and trends in the data.
5. Model Development: Model development is the process of building and training machine learning models using datasets to predict outcomes or make decisions. It involves selecting appropriate algorithms, tuning hyperparameters, and evaluating model performance.
6. Error Rates: Error rates refer to the frequency of inaccuracies or discrepancies in AI model predictions compared to ground truth or expected outcomes. High error rates can indicate poor model performance and may result from data quality issues or algorithmic deficiencies.
Now, let’s delve deeper into how automated data quality profiling can accelerate AI workflows and drive innovation across various domains.
In the realm of artificial intelligence (AI), the quality of data used for training and inference is paramount. Poor data quality can lead to inaccurate models, reduced performance, and unreliable predictions, hindering the potential of AI systems to deliver meaningful insights and value.
However, manually assessing and improving data quality can be time-consuming and resource-intensive. This is where automated data quality profiling comes into play, offering a powerful solution to accelerate AI by streamlining the process of data preparation and ensuring that the data used for AI applications is of high quality.
In this comprehensive guide, we’ll explore how automated data quality profiling can turbocharge AI workflows and drive innovation across various domains.
Efficient Data Preparation
Automated data quality profiling streamlines the process of data preparation by quickly and comprehensively analyzing datasets for inconsistencies, missing values, outliers, and other anomalies. This automation reduces the time and effort required for data cleaning and preprocessing, allowing data scientists and ML engineers to focus on higher-value tasks such as feature engineering and model development.
Improved Data Understanding
By providing insights into the characteristics and distribution of data, automated data quality profiling helps data scientists gain a deeper understanding of the dataset’s structure, patterns, and relationships. Visualizing data quality metrics and summary statistics enables data scientists to identify data quality issues more effectively and make informed decisions about feature selection, engineering, and transformation.
Faster Model Development
High-quality data is essential for building accurate and reliable AI models. Automated data quality profiling ensures that the data used for model training is clean, consistent, and representative of the underlying population. By detecting and addressing data quality issues early in the model development process, data scientists can accelerate model training and achieve better performance outcomes.
Reduced Error Rates
Poor data quality can lead to errors and inaccuracies in AI models, undermining their predictive capabilities and reliability. Automated data quality profiling tools can flag potential data quality issues proactively, allowing data scientists to take corrective actions and mitigate the risk of errors before deploying models into production. By minimizing error rates, automated data quality profiling enhances the trustworthiness and effectiveness of AI systems.
Enhanced Model Deployment
Automated data quality profiling streamlines the process of model deployment by ensuring that the input data meets the required quality standards. By integrating data quality checks into deployment pipelines, organizations can automate the validation of incoming data streams and trigger alerts or notifications when data quality issues are detected. This enables organizations to deploy AI models with confidence, knowing that they are operating on reliable and trustworthy data.
Continuous Monitoring and Improvement
Automated data quality profiling enables organizations to implement continuous monitoring mechanisms that track data quality metrics over time. By establishing baseline metrics and setting threshold values for acceptable data quality levels, organizations can detect deviations and anomalies in real-time and take proactive measures to maintain data quality. This iterative feedback loop ensures that AI systems remain effective and reliable as data evolves and changes over time.
Automated data quality profiling is a game-changer for accelerating AI workflows and driving innovation across various domains. By automating data quality assessment and validation processes, organizations can unlock the full potential of AI and harness its transformative power to deliver meaningful insights and value. With automated data quality profiling, the journey from data to AI becomes faster, more efficient, and more reliable, paving the way for smarter, more impactful AI applications in the future.