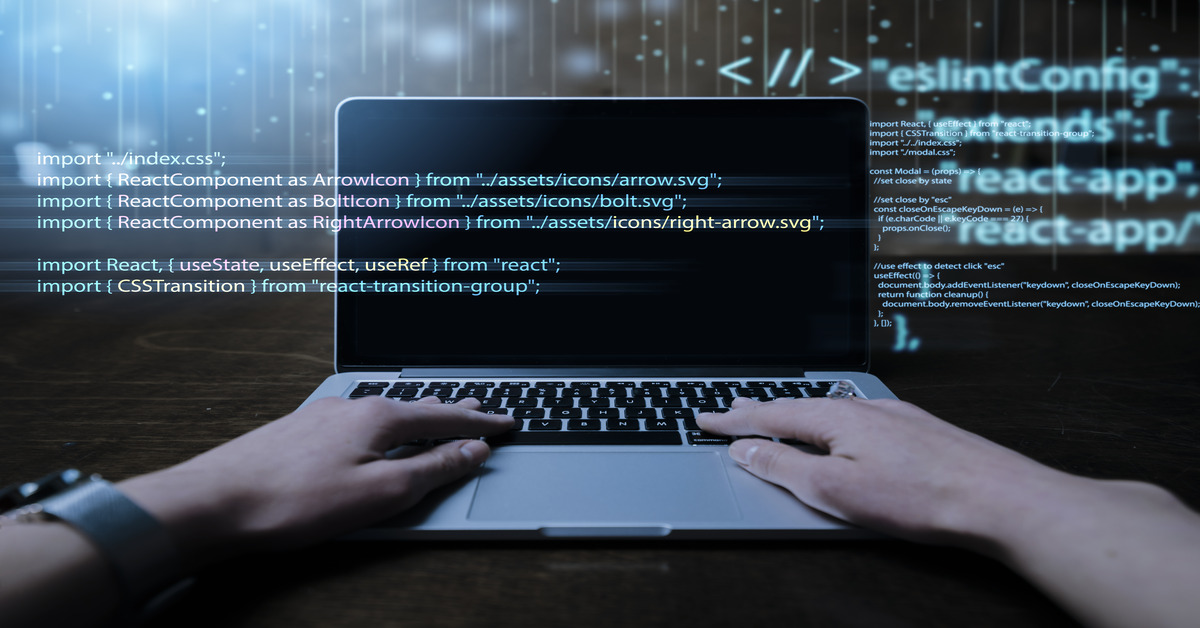
Summary: Master how to tabulate data in Python using essential libraries like Pandas and NumPy. This guide covers basic and advanced techniques, including handling missing data, multi-indexing, and creating pivot tables, enabling efficient Data Analysis and insightful decision-making.
Introduction
In Data Analysis, mastering how to tabulate data in Python is akin to wielding a powerful tool for extracting insights. This article offers a concise yet comprehensive overview of this essential skill. Analysts and Data Scientists can efficiently organise and structure raw information by tabulating data, paving the way for deeper analysis and visualisation.
Understanding the significance of tabulation lays the foundation for effective decision-making, enabling professionals to uncover patterns, trends, and correlations within datasets. Join us as we delve into the intricacies of data tabulation in Python, unlocking its potential for informed insights and impactful outcomes.
Getting Started with Data Tabulation Using Python
Tabulating data is a fundamental aspect of Data Analysis and is crucial in deriving insights and making informed decisions. With Python, a versatile and powerful programming language, you can efficiently tabulate data from various sources and formats.
Whether working with small-scale datasets or handling large volumes of information, Python offers robust tools and libraries to streamline the tabulation process. Understanding the basics is essential when tabulating data using Python. In this section, we’ll delve into the foundational concepts of data tabulation and explore how Python facilitates this task.
Basic Data Structures for Tabulation
Before diving into data tabulation techniques, it’s crucial to grasp the basic data structures commonly used in Python. These data structures are the building blocks for effectively organising and manipulating data. The primary data structures for tabulation include lists, dictionaries, and data frames.
Lists: Lists are versatile data structures in Python that allow you to store and manipulate sequences of elements. They can contain heterogeneous data types and are particularly useful for tabulating small-scale datasets.
Dictionaries: Dictionaries are collections of key-value pairs that enable efficient data storage and retrieval. They provide a convenient way to organise tabulated data, especially when dealing with structured information.
DataFrames: These are a central data structure in libraries like Pandas, offering a tabular data format similar to a spreadsheet or database table. DataFrames provide potent tools for tabulating and analysing data, making them a preferred choice for many Data Scientists and analysts.
Overview of Popular Python Libraries for Data Tabulation
Python boasts a rich ecosystem of libraries specifically designed for data manipulation and analysis. Two popular libraries for data tabulation are Pandas and NumPy.
Pandas: It is a versatile and user-friendly library that provides high-performance data structures and analysis tools. Pandas offers a DataFrame object and a wide range of functions for reading, writing, and manipulating tabulated data efficiently.
NumPy: It is a fundamental library for Python numerical computing. It provides support for large, multidimensional arrays and matrices. While not explicitly designed for tabulation, NumPy’s array-based operations are often used for data manipulation tasks with other libraries.
By familiarising yourself with these basic data structures and popular Python libraries, you’ll be well-equipped to embark on your journey into data tabulation using Python.
Tabulating Data with Pandas
Pandas is a powerful Python library widely used for data manipulation and analysis. This section will delve into the fundamentals of tabulating data with Pandas, covering everything from installation to advanced operations.
Installing and Importing Pandas
Before tabulating data with Pandas, you must install the library on your system. Installation is typically straightforward using Python’s package manager, pip. Open your command-line interface and execute the following command:
Once Pandas is installed, you can import it into your Python scripts or notebooks using the `import` statement:
Reading Data into Pandas DataFrame
Pandas provide various functions for reading data from different file formats such as CSV, Excel, SQL databases, etc. One of the most commonly used functions is `pd.read_csv()` for reading data from a CSV file into a Pandas DataFrame:
You can replace `’data.csv’` with the path to your CSV file. Pandas automatically detect the delimiter and other parameters to load the data correctly.
Basic DataFrame Operations for Tabulation
Once your data is loaded into a data frame, you can perform various operations to tabulate and manipulate it. Some basic operations include:
Selecting Data: Use square brackets `[]` or the `.loc[]` and `.iloc[]` accessors to select specific rows and columns.
Filtering Data: Apply conditional statements to filter rows based on specific criteria using boolean indexing.
Sorting Data: Use the `.sort_values()` method to sort the DataFrame by one or more columns.
Grouping and Aggregating Data with Pandas
Grouping and aggregating data are essential techniques for summarising and analysing datasets. Pandas provides the `.groupby()` method for grouping data based on one or more columns. After grouping, you can apply aggregation functions such as `sum()`, `mean()`, `count()`, etc., to calculate statistics for each group.
This code groups the DataFrame `df` by the ‘category’ column. It calculates the sum of the ‘value’ column for each group.
Mastering these basic operations with Pandas is crucial for efficient data tabulation and analysis in Python.
Advanced Techniques for Data Tabulation
Mastering data tabulation involves more than just basic operations. Advanced techniques can significantly enhance your data manipulation and analysis capabilities. This section explores how to handle missing data, perform multi-indexing, create pivot tables, and combine datasets for comprehensive tabulation.
Handling Missing Data in Tabulated Datasets
Missing data is a common issue in real-world datasets, and how you handle it can significantly affect your analysis. Python’s Pandas library provides robust methods to manage missing data effectively.
First, identify missing data using the `isnull()` function, which helps locate NaNs in your DataFrame. You can then decide whether to remove or impute these values. Use `dropna()` to eliminate rows or columns with missing data. This method is straightforward but might lead to a significant data loss.
Alternatively, the `fillna()` method can fill missing values. This function allows you to replace NaNs with specific values, such as the mean and median, or a technique such as forward-fill or backward-fill. Choosing the right strategy depends on your dataset and analysis goals.
Performing Multi-Indexing and Hierarchical Tabulation
Multi-indexing, or hierarchical indexing, enables you to work with higher-dimensional data in a structured way. This technique is invaluable for managing complex datasets containing multiple information levels.
In Pandas, create a multi-index DataFrame by passing a list of arrays to the `set_index()` method. This approach allows you to perform operations across multiple levels. For instance, you can aggregate data at different levels using the `groupby()` function. Multi-indexing enhances your ability to navigate and analyse data hierarchically, making it easier to extract meaningful insights.
Pivot Tables for Advanced Data Analysis
Pivot tables are potent tools for summarising and reshaping data, making them ideal for advanced Data Analysis. You can create pivot tables in Python using Pandas `pivot_table()` function.
A pivot table lets you group data by one or more keys while applying an aggregate function, such as sum, mean, or count. This functionality simplifies data comparison and trend identification across different dimensions. By specifying parameters like `index`, `columns`, and `values`, you can customise the table to suit your analysis needs.
Combining and Merging Datasets for Comprehensive Tabulation
Combining and merging datasets is essential when dealing with fragmented data sources. Pandas provides several functions to facilitate this process, including `concat()`, `merge()`, and `join()`.
Use `concat()` to append or stack DataFrames vertically or horizontally. This function helps add new data to an existing dataset. Like SQL joins, the `merge()` function combines datasets based on standard columns or indices. This method is perfect for integrating related data from different sources. The `join()` function offers a more straightforward way to merge datasets on their indices, simplifying the combination process.
These advanced techniques can enhance your data tabulation skills, leading to more efficient and insightful Data Analysis.
Tips and Tricks for Efficient Data Tabulation
Efficient data tabulation in Python saves time and enhances the quality of your Data Analysis. Here, we’ll delve into some essential tips and tricks to optimise your data tabulation process.
Utilising Vectorised Operations for Faster Tabulation
Vectorised operations in Python, particularly with libraries like Pandas and NumPy, can significantly speed up data tabulation. These operations allow you to perform computations on entire arrays or DataFrames without explicit loops.
You can leverage the underlying C and Fortran code in these libraries using vectorised operations, much faster than Python’s native loops. For instance, consider adding two columns in a DataFrame. Instead of using a loop to iterate through each row, you can simply use:
This one-liner makes your code more concise and drastically reduces execution time. Embrace vectorisation whenever possible to maximise efficiency.
Optimising Memory Usage When Working with Large Datasets
Large datasets can quickly consume your system’s memory, leading to slower performance or crashes. Optimising memory usage is crucial for efficient data tabulation.
One effective approach is to use appropriate data types for your columns. For instance, if you have a column of integers that only contains values from 0 to 255, using the `int8` data type instead of the default `int64` can save substantial memory. Here’s how you can optimise a DataFrame:
Additionally, consider using chunking techniques when reading large files. Instead of loading the entire dataset at once, process it in smaller chunks:
This method ensures you never exceed your memory capacity, maintaining efficient data processing.
Customising Tabulated Output for Readability and Presentation
Presenting your tabulated data is as important as the analysis itself. Customising the output can enhance readability and make your insights more accessible.
Start by formatting your DataFrame using Pandas’ built-in styling functions. You can highlight important data points, format numbers, and even create colour gradients. For example:
Additionally, when exporting data to formats like CSV or Excel, ensure that headers and index columns are appropriately labelled. Use the `to_csv` and `to_excel` methods with options for customisation:
These small adjustments can significantly improve the presentation quality of your tabulated data.
Leveraging Built-in Functions and Methods for Streamlined Tabulation
Python libraries offer many built-in functions and methods that simplify and expedite the tabulation process. Pandas, in particular, provide powerful tools for data manipulation.
For instance, the `groupby` method allows you to group data by specific columns and perform aggregate functions such as sum, mean, or count:
Similarly, the `pivot_table` method lets you create pivot tables, which are invaluable for summarising and analysing large datasets:
Mastering these built-in functions can streamline your data tabulation workflow, making it faster and more effective.
Incorporating these tips and tricks into your data tabulation process will enhance efficiency, optimise resource usage, and improve the clarity of your presented data, ultimately leading to more insightful and actionable analysis.
Read More:
Data Abstraction and Encapsulation in Python Explained.
Anaconda vs Python: Unveiling the differences.
Frequently Asked Questions
What Are The Basic Data Structures For Tabulating Data In Python?
Lists, dictionaries, and DataFrames are the primary data structures for tabulating data in Python. Lists store sequences of elements, dictionaries manage key-value pairs, and DataFrames, available in the Pandas library, offer a tabular format for efficient Data Analysis.
How Do You Handle Missing Data In Tabulated Datasets Using Python?
To manage missing data in Python, use Pandas’ `isnull()` to identify NaNs. Then, use `dropna()` to remove them or `fillna()` to replace them with appropriate values like the mean or median, ensuring data integrity.
What Are Some Advanced Techniques For Data Tabulation In Python?
Advanced tabulation techniques in Python include handling missing data, performing multi-indexing for hierarchical data, creating pivot tables for summarisation, and combining datasets using functions like `concat()`, `merge()`, and `join()` for comprehensive Data Analysis.
Conclusion
Mastering how to tabulate data in Python is essential for Data Analysts and scientists. Professionals can efficiently organise, manipulate, and analyse data by understanding and utilising Python’s powerful libraries, such as Pandas and NumPy.
Techniques like handling missing data, multi-indexing, and creating pivot tables enhance the depth of analysis. Efficient data tabulation saves time and optimises memory usage, leading to more insightful and actionable outcomes. Embracing these skills will significantly improve data-driven decision-making processes.