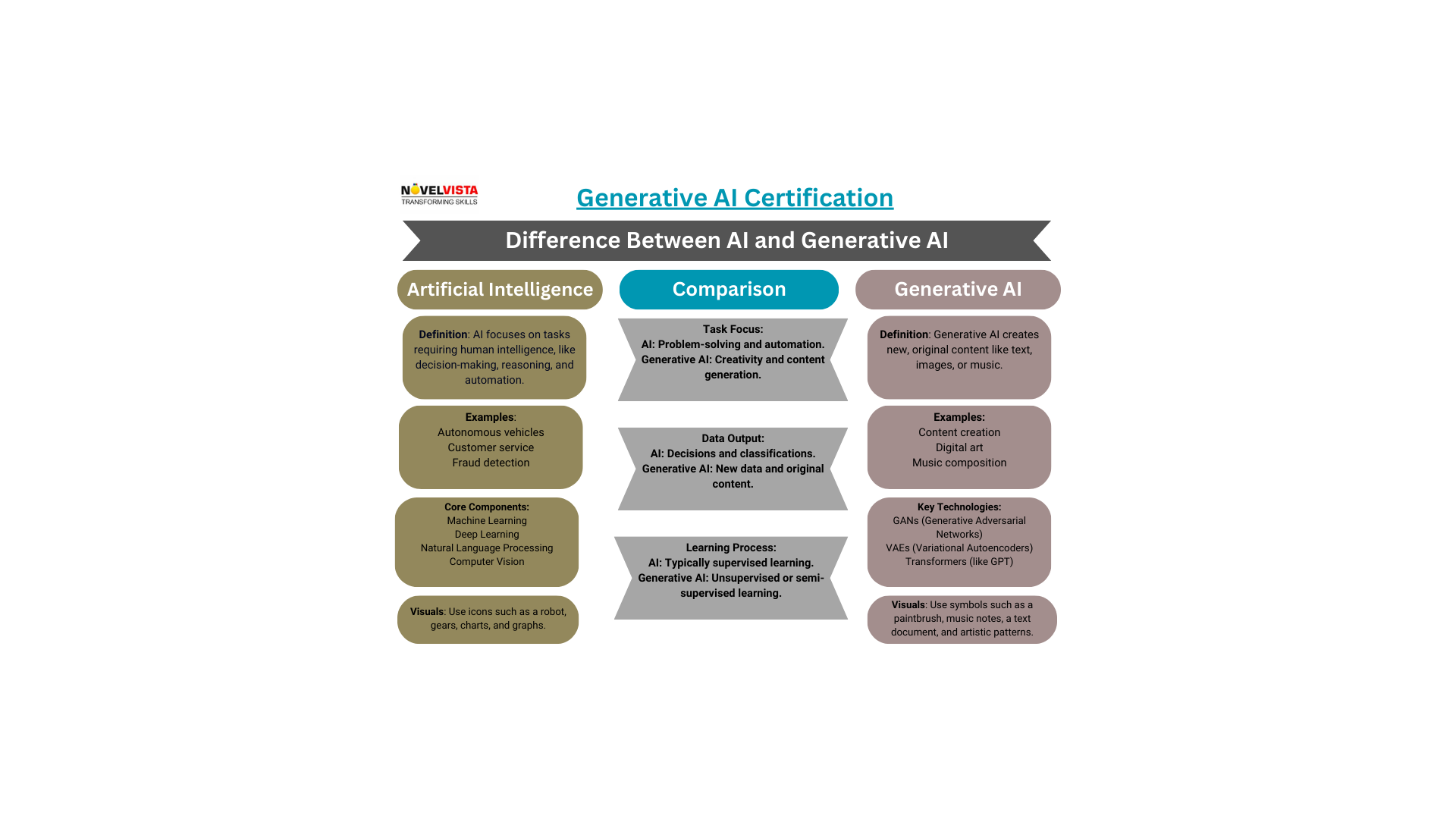
Artificial Intelligence (AI) has become one of the most transformative technologies of our era, revolutionizing industries and reshaping how we live, work, and interact. In variety of applications AI can found like, from autonomous vehicles to smart personal assistants like Siri and Alexa. Among the many branches of AI, Generative AI has recently gained significant attention due to its ability to create new Art, original content. While AI and Generative AI are closely related, they have distinct features, applications, and mechanisms that differentiate them.
Nowadays growing demand for expertise in this field therefore, obtaining a Generative AI Certification is becoming increasingly valuable for professionals looking to specialize in this cutting-edge technology.
In this article, we will explore the key differences between AI and Generative AI, examining their fundamental principles, how they function, and their impact across different industries.
What is Artificial Intelligence?
Artificial Intelligence (AI) is the broad field of computer science that focuses on creating machines or systems capable of performing tasks that traditionally require human intelligence. AI systems aim to mimic cognitive functions such as learning, reasoning, problem-solving, decision-making, language processing, and perception. These tasks are generally complex and require a combination of computational power and algorithms to function effectively.
AI can be categorized into two major types:
- Narrow AI (Weak AI):
- Narrow AI refers to systems designed for a specific task or a narrow range of tasks. These systems are not conscious and cannot perform functions outside of their designated tasks. Examples of narrow AI include spam filters, image recognition software, and recommendation algorithms used in platforms like Netflix or Amazon.
- General AI (Strong AI):
- General AI is a more advanced form of AI that can understand, learn, and apply knowledge across different domains, similar to human intelligence. While general AI is still largely theoretical, it is the long-term goal of AI research to build systems that can perform any intellectual task that a human can.
Core Components of AI
AI systems are driven by a combination of several components and techniques, including:
- Machine Learning (ML): A subset of AI that enables systems to learn from data and improve over time without being explicitly programmed. This involves training models using large datasets.
- Deep Learning (DL): A subset of machine learning that uses neural networks with many layers (hence the term “deep”) to perform tasks such as image and speech recognition.
- Natural Language Processing (NLP): A branch of AI focused on the interaction between computers and human languages, enabling machines to understand, interpret, and generate human language.
- Computer Vision: A field within AI that allows machines to interpret and make decisions based on visual data, such as images or videos.
Applications of AI
AI has found applications across virtually every industry, from healthcare to finance, manufacturing, and entertainment. Some common AI applications include:
- Healthcare: AI-powered systems can diagnose diseases, analyze medical images, and even assist in surgeries.
- Finance: AI helps in detecting fraud, automating trading, and managing risk in financial markets.
- Autonomous Vehicles: AI algorithms are essential for enabling self-driving cars to navigate roads safely.
- Customer Service: AI chatbots provide automated, round-the-clock support for customer inquiries.
What is Generative AI?
Generative AI, as the name suggests, refers to a type of artificial intelligence that can generate new content. This content can take various forms, such as text, images, audio, video, or even 3D models. What distinguishes Generative AI from other types of AI is its ability to create new, original material rather than simply recognizing patterns, categorizing data, or performing pre-programmed tasks.
Generative AI Course can provide essential skills, helping creatives leverage AI tools to push the boundaries of their craft and address the unique opportunities and challenges presented by AI-driven media.
is a class of models known as Generative Models. These models, trained on vast amounts of data, can generate new outputs that resemble the data they were trained on. Generative AI techniques include:
- Generative Adversarial Networks (GANs): GANs consist of two neural networks — one that generates content (the generator) and one that evaluates the generated content (the discriminator). Through an iterative process, the generator learns to create more convincing outputs, while the discriminator gets better at distinguishing between real and fake data.
- Variational Autoencoders (VAEs): VAEs are a type of neural network that compresses data into a lower-dimensional space (latent space) and then reconstructs it. They are used for generating new data that closely resembles the input data.
- Transformers: These are a more recent advancement in generative AI, especially for text-based tasks. Models like Open AI’s GPT (Generative Pre-trained Transformer) and Google’s BERT are examples of transformers used to generate human-like text.
Applications of Generative AI
Generative AI is already having a profound impact on various industries, particularly in creative fields. Some common applications include:
- Content Creation: Generative AI is used to produce written content, such as articles, poems, or even entire books. For example, GPT models are capable of generating coherent, context-aware text.
- Art and Design: Generative AI can create stunning visuals, artwork, or designs. Tools like DALL-E, which can generate images from text descriptions, are making waves in the art world.
- Music: AI models can compose original pieces of music or remix existing songs, opening up new possibilities for musicians and composers.
- Game Development: In video game design, generative AI can create levels, characters, or even narratives, saving developers significant time and effort.
- Synthetic Data Generation: Generative AI can create synthetic data for training machine learning models when real-world data is scarce or expensive to collect.
Key Differences Between AI and Generative AI
While Generative AI is a subset of AI, there are notable differences in how they function and what they aim to achieve:
- Task Focus vs. Creativity:
- Traditional AI is generally designed for problem-solving and automation, focusing on tasks like prediction, classification, and optimization. Generative AI, on the other hand, focuses on creativity, producing new content based on the patterns and information it has learned.
2.Data Output:
- In conventional AI, the output is often a decision, classification, or recommendation based on input data. For example, an AI-powered recommendation engine suggests movies based on user preferences. Generative AI, however, creates entirely new data — such as a painting, story, or video — that did not exist before.
3.Learning Process:
- Many AI systems rely on supervised learning, where they are trained with labelled data. Generative AI typically employs unsupervised or semi-supervised learning, where the model learns the patterns and distributions in the data without being explicitly told what to generate.
4.Use Cases:
- AI applications are found in areas requiring analysis and decision-making, like financial fraud detection or autonomous driving. Generative AI shines in domains where original content creation is required, like digital art, entertainment, and personalized content generation.
Conclusion
Both AI and Generative AI represent groundbreaking advancements in technology, but they serve different purposes. AI is a broad field encompassing various technologies aimed at solving tasks that require intelligence, while Generative AI specifically focuses on the creation of new, original content. As these technologies continue to evolve, their applications will become more integrated into our daily lives, offering new possibilities for automation, creativity, and innovation across industries.