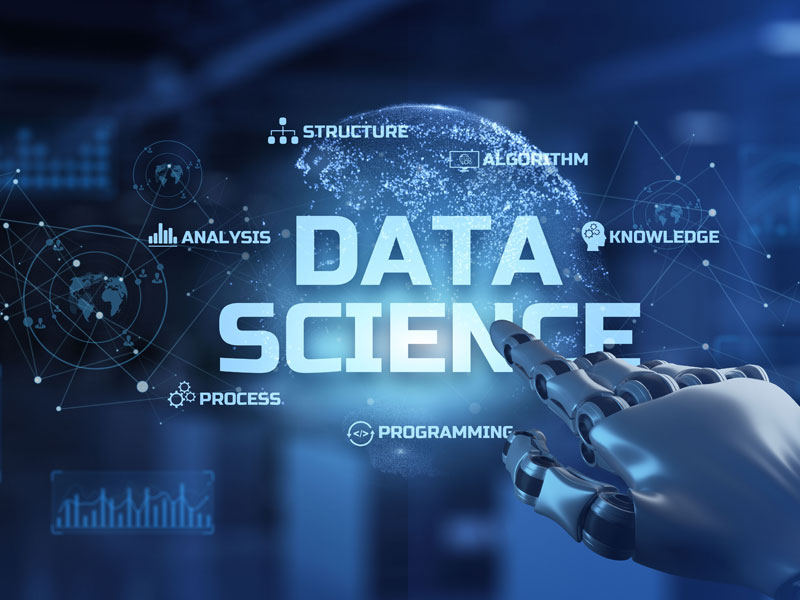
Information is king in the data-driven world of today. However, unprocessed raw data is not very valuable. To help businesses make wise decisions and accomplish their strategic objectives, data scientists may be thought of as translators and interpreters who take in and extract knowledge and insights. You can opt for the Best Data Science Training in Gurgaon, Delhi, Pune, Noida and other parts of India.
What does a data scientist perform, though? A data scientist is responsible for a broad variety of tasks that need a special combination of technical expertise, analytical talent, and commercial sense. Here, we dive into the complex field of data science and examine the fundamental duties and jobs that make up this important industry.
1. Data Acquisition and Wrangling: The Art of Gathering and Refining
Data is the starting point of a data scientist’s journey. They are in charge of locating pertinent data sources, which may include social media feeds, corporate databases, consumer surveys, or sensor readings from Internet of Things devices. Data scientists locate this information, extract it, and verify its completeness and correctness.
However, data is rarely perfect. It could be erroneous, conflicting, or lacking in important details. This is when data wrangling becomes important. The data is cleaned and preprocessed by scientists, who also fix errors and duplication and format it so that it may be used for research. For wrangling and manipulating data, programs like Python and SQL are helpful.
2. Exploratory Data Analysis (EDA): Unveiling the Secrets Within
The first investigative task in data science is called exploratory data analysis (EDA). Here is when data scientists get their hands dirty—they delve into unprocessed data to find hidden correlations and patterns. They gain a thorough grasp of the data by employing methods like statistical analysis and data visualization, which enable them to spot trends, outliers, and possible links.
Imagine it as a detective searching a crime scene for hints; however, in this instance, the hints point to important information that is just waiting to be discovered. The foundation for developing models and obtaining information that can help with improved decision-making is laid by this preliminary investigation.
3. Building Predictive Models: From Insights to Actionable Strategies
After identifying trends and connections, data scientists build models to generate forecasts. Their proficiency with statistics and machine learning is evident here. They choose and put into practice methods that can learn from past data and forecast future occurrences, such as deep learning models, decision trees, and linear regression.
A data scientist may create a model, for example, that forecasts customer attrition, which would enable a business to target at-risk clients with retention initiatives. A thorough evaluation of the model’s performance guarantees its correctness and generalizability.
4. Communication and Storytelling: Translating Data into Action
Data scientists are interpreters of a complicated language, not just mathematicians. The magic occurs once they’ve gleaned insights from unprocessed data. They use direct and succinct communication to turn boring numbers into gripping stories. Consider them to be data whisperers who can translate obscure truths into compelling narratives.
They provide both expert and non-technical audiences with a clear image through presentations, reports, and data visualizations. Since it serves as the link between information and action, this narrative skill is essential. Insights can only be implemented into practical methods that promote positive change inside businesses when they strike a chord.
5. Collaboration: The Power of Teamwork
Usually, data science is a lonesome endeavour. Data scientists frequently operate in teams, coordinating with software engineers who integrate models into apps, business analysts who convert business demands into data-driven inquiries, and data engineers who create and manage data pipelines. Successful implementation of data-driven solutions requires excellent cooperation and communication.
6. Staying Abreast of the Curve: Continuous Learning
Although data is a powerful tool, it must be used responsibly, just like any other instrument. Data scientists take on the role of guardians, making sure that moral issues are integrated into everything they do. They keep a close eye out for The field of data science and operate in a dynamic environment where new technologies and developing algorithms are always changing the game.
Data scientists are lifelong learners who constantly improve their skills in order to stay ahead of the curve. By consuming online courses, going to conferences, and immersing themselves in the most recent research, they actively seek out fresh information. This ongoing learning is about developing a growth mindset, accepting change, and remaining flexible in addition to gaining new abilities.
Data scientists make sure they stay current in this ever-changing industry by never stopping studying, and being able to use the newest instruments and methods to extract
7. Ethical Considerations: Responsible Use of Data
Although data is a powerful tool, it must be used responsibly, just like any other instrument. Data scientists take on the role of guardians, making sure that moral issues are integrated into everything they do.
They actively search for biases in datasets and algorithms in an effort to reduce them and stop discriminating results. Regulations regarding data privacy are another important factor. Data scientists make sure that regulations protecting personal information are followed by navigating a challenging legal environment. This moral compass makes sure that information is used for the greater good of society by encouraging justice, openness, and responsible decision-making.
8. Beyond Core Skills: The Well-Rounded Data Scientist
Data science is more than just using sophisticated algorithms. Beyond technical proficiency, the most prosperous practitioners have a special toolkit. Their insatiable curiosity drives their investigation, and their ability to think critically helps them make sense of the complicated facts.
As they go through disorganised information and uncover buried facts, problem-solving becomes second nature to them. The real covert weapon, though? Business intelligence. They are able to translate data insights into real business value because they speak the same language as the enterprise. They are tremendous assets because of their capacity to connect the technological and business domains, enabling data-driven decision-making that advances enterprises.
The Diverse Landscape of Data Science Roles
The particular duties and obligations of a data scientist might change based on the project specifications, organization size, and industry. An overview of some of the popular specialties in data science is provided below:
- Data Analyst: concentrates on gathering, preparing, and analyzing data; it frequently offers answers to certain business queries.
- Machine Learning Engineer: specializes in creating, implementing, and managing models for machine learning.
- Data Architect: creates and manages the infrastructure for data that facilitates the gathering, storing, and analysis of data.
- Business Intelligence Analyst: connects data science and business, converting ideas into practical.
Conclusion
A data scientist’s roles and responsibilities include analyzing complex data sets to extract actionable insights, building and implementing predictive models, and interpreting results to inform business decisions. They collaborate with stakeholders to understand business needs, develop data-driven strategies, and communicate findings effectively. Their work combines statistical analysis, programming, and domain expertise to drive innovation and optimize processes.